Artificial Intelligence and Phone Collection: assigning the best agents to the most complex practices
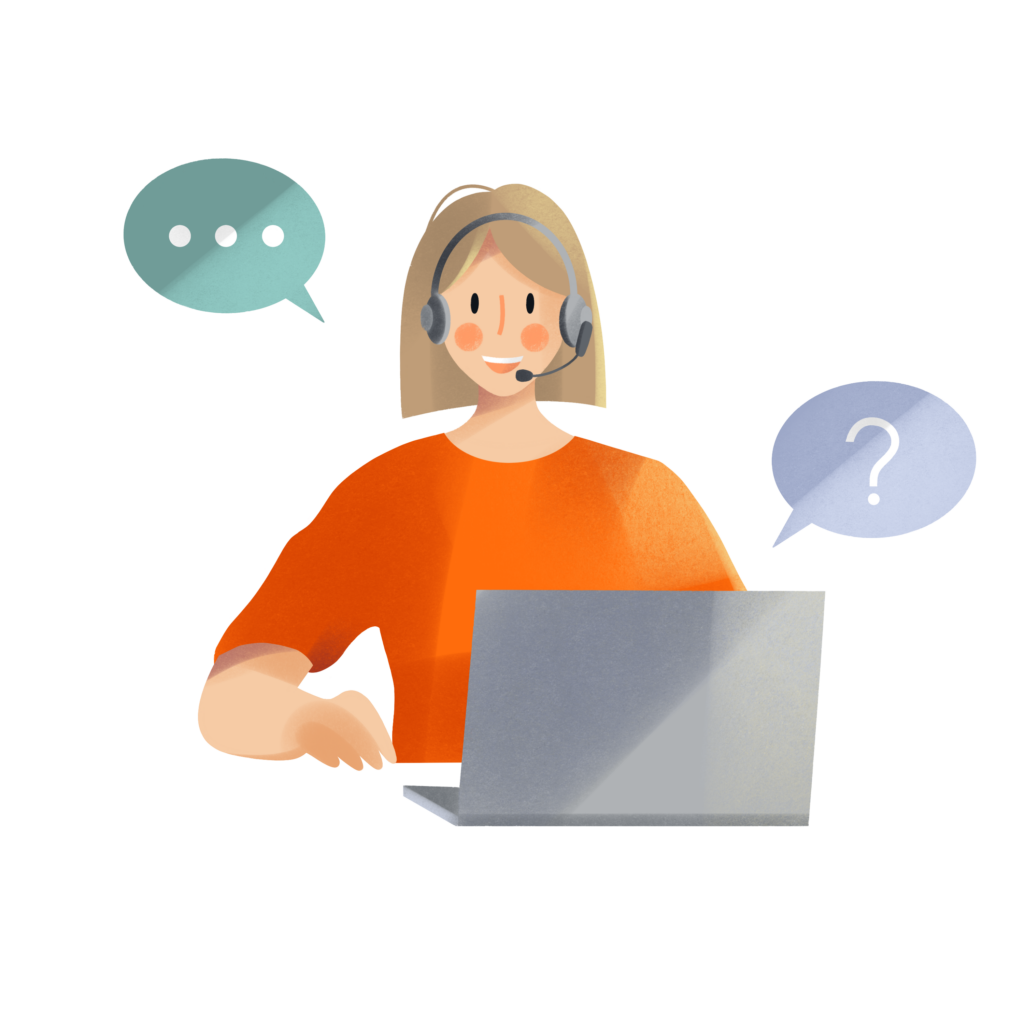
Debt collection campaigns can be very complicated to manage and the companies that manage them must take into account numerous variables in order to achieve their objectives, such as the propensity of a debtor to repay their debt and the economic value that has to be recovered.
Currently, the processing method used by most companies dealing with Debt Collection consists of randomly assigning the contacts present in the lists to the operators, without performing any prior analysis on them.
For example, a company that manages Phone Collection campaigns for a Customer in the Utilities sector and that initially contacts 10,000 positions per month, will proceed to randomly assign each of these contacts to the operators. This means that many of the most complex positions to recover will be handled by inexperienced operators, at the risk of missing several recovery opportunities. In this way, the achievement of the recovery objective is tied to random factors that, if not identified, significantly reduce the company’s chances of achieving its objectives.
In order to be able to define in advance a strategy that is capable of ensuring the achievement of the objective quickly, it is therefore necessary to carry out an in-depth study of the lists of debtors in order to identify in advance which are, among the different positions, the most difficult and the easiest to recover.
In this way, the most difficult debts to recover will be assigned to the most skilled and veteran operators, thus maximizing the chances of recovery. The simpler positions, on the other hand, will be entrusted to less experienced operators, who will be able to practice and thus learn the best strategies to successfully complete a recovery action.
But how is it possible to analyse debtors in advance to strategically assign them to operators?
Thanks to BigProfiles, the first Artificial Intelligence Platform for Debt Collection, debt collection companies can analyse the positions on the list and predict the propensity to recover for each one of them, in order to assign the most complex ones to the more experienced operators. In this way, it is possible to increase the total number of recoveries made by up to 20%, in the same amount of time that it would take to work through the lists randomly.
Do you want to know more about how to implement BigProfiles within your company? Request a free demo on our websitewww.bigprofiles.com!