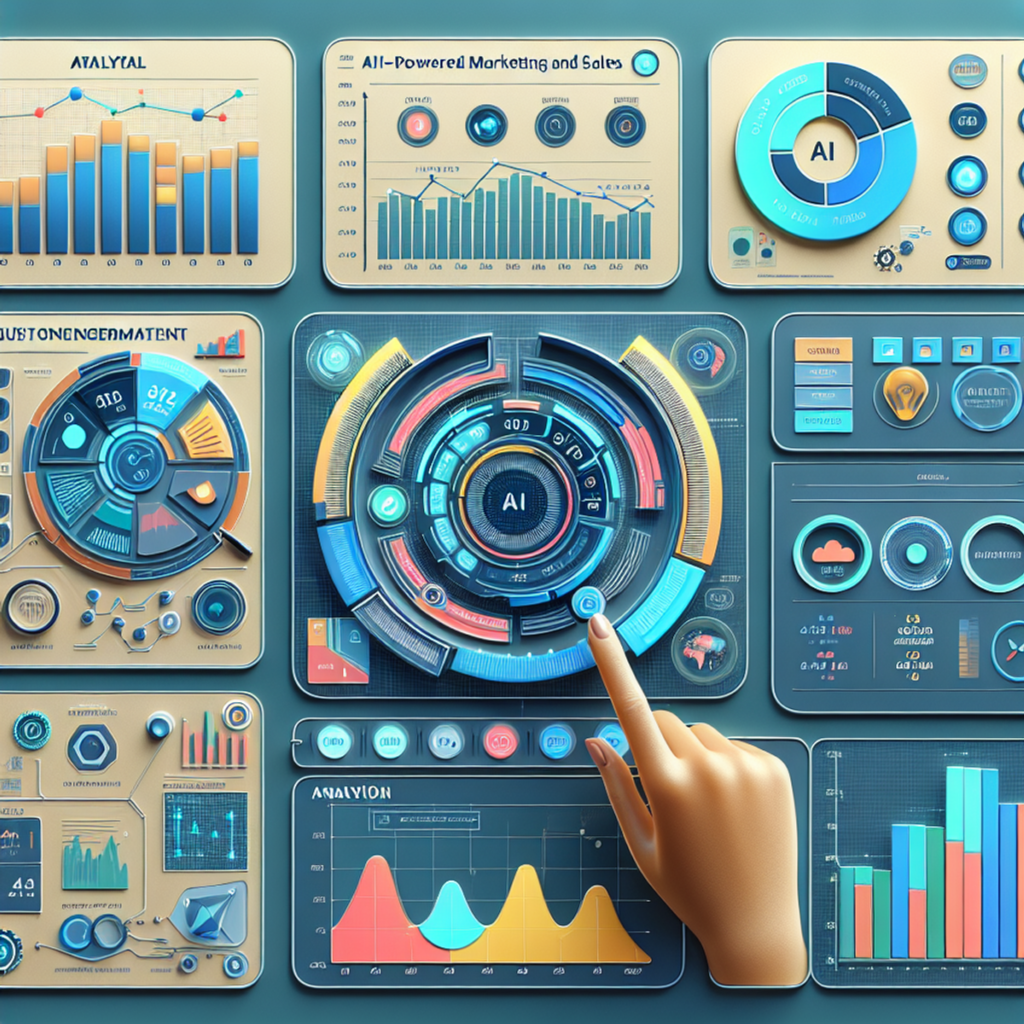
What is AI Marketing and How Do Predictive Models Work
AI marketing represents a revolution in the way companies interact with their customers. By using artificial intelligence, businesses can analyze large volumes of data to identify patterns and trends, thus improving the personalization and effectiveness of marketing campaigns.
Predictive Models
Predictive models play a crucial role in predicting customers’ future actions. These models use advanced algorithms to analyze historical data and identify recurring behaviors. Two fundamental components are:
- Data Analytics: The systematic collection and analysis of data that provide detailed insights into customer behavior.
- Machine Learning: Algorithms that learn from past data to make accurate predictions about future events.
Connection between Data Analytics, Machine Learning, and Predictive Models
In the field of AI marketing, there is a close connection between data analytics, machine learning, and predictive models. This synergy allows for:
- Identifying Customer Segments: Distinguishing customer groups based on their behaviors and preferences.
- Predicting Churn: Using predictive models to anticipate which customers might abandon the service.
- Optimizing Marketing Campaigns: Creating highly personalized messages that resonate with specific customer segments.
Through these technologies, companies can make data-driven decisions, significantly improving their marketing strategies.
Churn Prediction in Marketing: Definition and Importance
Churn prediction, or churn prediction, is the process of identifying customers who are more likely to stop using a product or service. This phenomenon represents a significant challenge for companies, as acquiring new customers is often more expensive than retaining existing ones.
Why It Matters
- Maintaining existing customers: Retaining current customers contributes to the financial stability of the company.
- Reducing acquisition costs: Focusing resources on customer retention strategies is generally more cost-effective than acquiring new customers.
- Increasing customer lifetime value (CLV): Loyal customers tend to spend more over time, increasing the overall CLV.
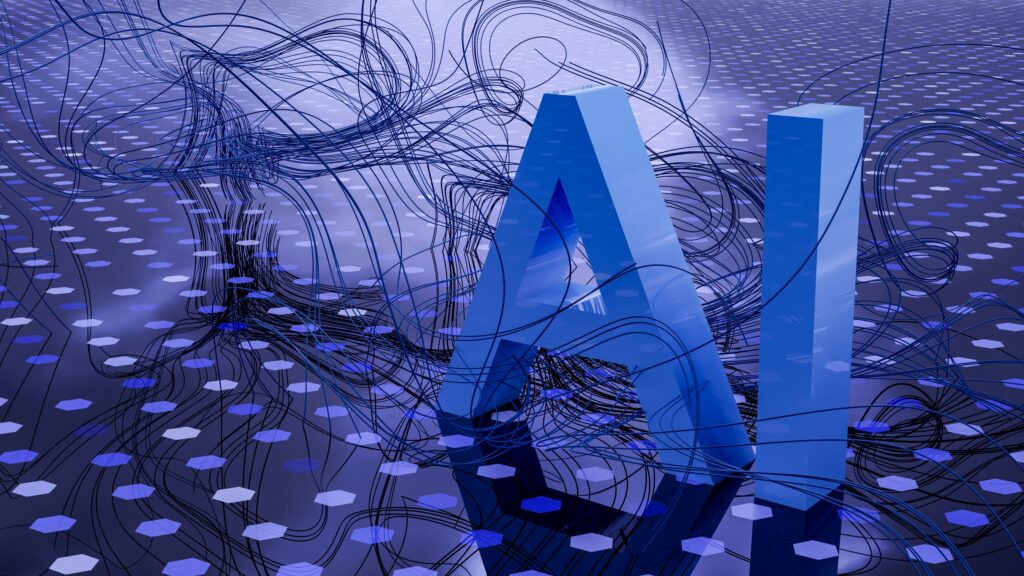
Role of AI-based Predictive Models
AI-based predictive models significantly improve the accuracy and effectiveness of churn prediction in marketing. Through advanced techniques such as machine learning, these models:
- They analyze large amounts of data: They collect and analyze historical customer data to identify behavioral patterns.
- They identify early warning signs: They detect subtle clues that may indicate potential churn.
- They optimize intervention strategies: They provide personalized recommendations to prevent customer loss.
For example, a predictive model can identify that customers who gradually reduce their service usage are at risk of churn. The company can then take proactive measures, such as targeted offers or service improvements, to retain these customers.
Using Predictive Models for Churn Prediction in Marketing
Predictive models offer different techniques to address churn prediction, each with its own characteristics and benefits.
Modeling Methods
- Time Series Forecasting: This technique analyzes historical data to identify patterns and trends that can predict future behavior. It is particularly useful for detecting seasonal or cyclical variations in customer behavior.
- Machine Learning: Machine learning algorithms, such as neural networks and decision trees, learn from historical data to make accurate churn predictions. These algorithms dynamically adapt to new data, continuously improving their effectiveness.
Concrete Examples
Leading companies in the industry apply these models successfully:
- E-commerce: By using machine learning algorithms, a company can predict which customers are more likely to not make further purchases. For example, Amazon uses predictive models to identify customers at risk of churn and send them personalized offers.
- Telecommunications: Telecom companies use time series forecasting to monitor customer service usage. For instance, Vodafone can identify customers who gradually reduce their service usage and intervene with targeted promotional packages.
These techniques allow companies to take preventive measures based on accurate predictions, thus improving customer retention and optimizing marketing strategies.
BigProfiles: The Best Software for Generating AI Predictive Models
BigProfiles is an innovative solution in the field of AI software, designed to help businesses create powerful predictive models. This platform leverages artificial intelligence to enable even those without specific data science skills to develop effective and accurate models.
Key Features of BigProfiles
- User-Friendly: Intuitive interface that simplifies the process of creating predictive models.
- Complete Automation: Automates data collection and analysis, significantly reducing the time required to obtain useful results.
- Simple Integration: Easily integrates with existing business systems, improving operational efficiency.
- Scalability: Adaptable to the needs of companies of different sizes, from small businesses to large corporations.
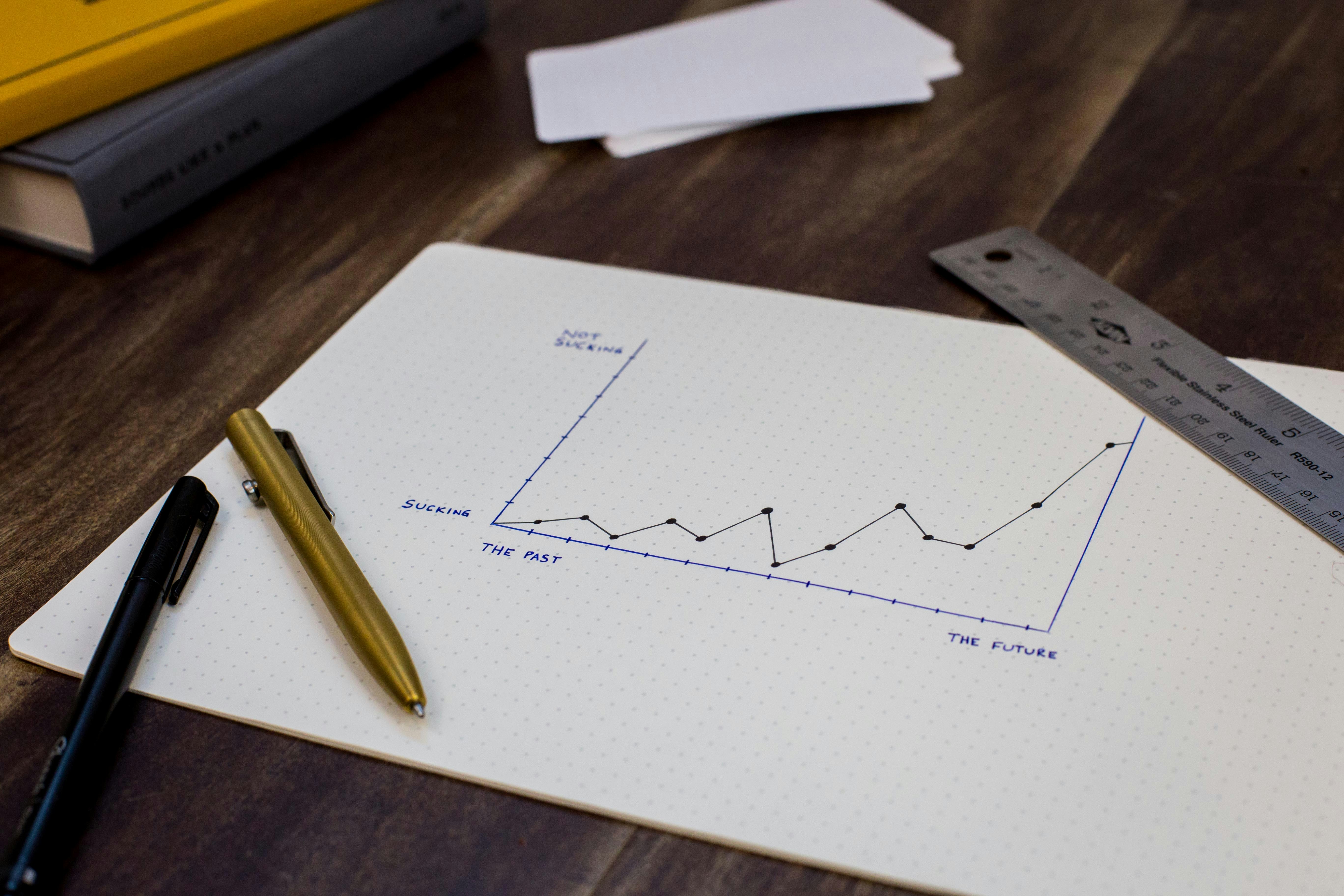
Distinctive Advantages
- Predictive Accuracy: Thanks to advanced machine learning algorithms, BigProfiles offers extremely accurate predictions regarding customer churn probabilities.
- Churn Reduction: By identifying at-risk customers in a timely manner, companies can implement targeted strategies for retention.
- Cost Savings: By optimizing resources and marketing efforts through precise forecasts, waste is reduced and return on investment is maximized.
BigProfiles represents a cutting-edge solution for those who want to fully exploit the potential of artificial intelligence in predictive marketing. This is also demonstrated by academic research that has highlighted the effectiveness of similar tools in the industry, as shown in this study on AI and predictive models, which confirms the numerous advantages offered by BigProfiles.
Practical Implementation of AI Predictive Models for Churn Prediction
Implementing predictive models for churn prediction requires a series of key phases. Each phase is crucial to ensure the accuracy and effectiveness of the model.
Project Definition
Establish clear and measurable objectives. Determine success metrics and define the specific problem of churn prediction that you intend to solve.
Data Collection and Processing
- Data Collection: Identify and gather relevant data from various touchpoints, such as transaction history, interactions with customer service, and online behaviors.
- Data Cleaning: Eliminate duplicate or inconsistent data. Make the data homogeneous to ensure that it is ready for analysis.
- Feature Engineering: Create new variables (features) that can improve the accuracy of the model, such as purchase frequency or time since the last transaction.
Creation and Validation of the Model
- Algorithm Selection: Choose suitable machine learning algorithms, such as decision trees, random forests, or neural networks.
- Model Training: Use a subset of historical data to train the model.
- Model Validation: Test the model on a separate dataset to evaluate its performance in terms of accuracy, recall, and F1 score.
Iteratively repeat these phases until obtaining an optimized model that can accurately predict churn-risk customers.
Advantages and Challenges in Using AI Predictive Models to Predict Churn
Advantages of Predictive Models
The effective use of AI predictive models to predict churn offers many significant advantages:
- Improvement of retention strategies: Predictive models allow the identification of customers at risk of churn well in advance, enabling companies to implement targeted strategies to retain them.
- Operational efficiency: By automating data analysis and churn prediction, companies can reduce operational costs and improve the efficiency of the marketing team.
- Personalization of offers: With a detailed understanding of customer behavior, companies can create personalized offers that better meet individual needs, thereby increasing customer satisfaction and loyalty.
Challenges and Ethical Considerations
The use of predictive models based on artificial intelligence also entails some challenges and ethical considerations:
- Data Quality: The accuracy of the models depends heavily on the quality and quantity of available data. Incomplete or incorrect data can compromise the accuracy of predictions.
- Bias in Models: Machine learning algorithms can reflect biases present in historical data, leading to unbalanced decisions. It is essential to monitor and correct such biases to ensure fairness.
- Data Privacy: The intensive use of personal data raises concerns about privacy. Companies must ensure compliance with all data protection regulations, such as GDPR.
These aspects highlight the need for a balanced approach that maximizes the benefits of predictive AI models while carefully addressing ethical and operational challenges.
Future Perspectives of AI Marketing and Predictive Models in Churn Prediction
AI marketing is rapidly changing, offering new opportunities to improve churn prediction. Some key developments and future trends include:
- Integration of new technologies: The combined use of AI with other emerging technologies such as the Internet of Things (IoT) and 5G can provide real-time data, improving the accuracy of predictive models.
- Enhancement of personalization: Advanced machine learning models can analyze more detailed individual behaviors, enabling highly personalized retention strategies.
- Adoption of self-learning predictive models: Systems that autonomously learn from data without human intervention can dynamically adapt to changes in customer behaviors.
- Ethics and transparency: With increasing concerns about privacy, companies will need to ensure that their predictive models are ethical and transparent, building trust with customers.
- Development of no-code/low-code platforms: Platforms like BigProfiles will make predictive models increasingly accessible even to those without specific technical skills.
These trends outline a future where the integration between AI and marketing will become increasingly sophisticated, leading to proactive churn prediction management.
Frequently Asked Questions
What is AI Marketing and what is its impact in the industry?
Brief introduction to AI in marketing and its impact.
How do Predictive Models work in the context of marketing?
Explanation of predictive models and the key role they play in predicting customers’ future actions in the context of marketing.
What is the connection between data analytics, machine learning, and predictive models in AI marketing?
Connection between data analytics, machine learning, and predictive models in the field of AI marketing.
Why is it crucial for companies to maintain existing customers and how can AI predictive models improve churn prediction?
Explanation of the churn prediction concept and why it is crucial for companies to maintain existing customers. How AI predictive models can significantly enhance the accuracy and effectiveness of churn prediction in the marketing context.
What methods and techniques are used to address the specific challenge of churn prediction?
Various modeling methods and techniques used to tackle the specific challenge of churn prediction, including approaches based on time series forecasting and machine learning algorithms.
How can BigProfiles help companies create powerful predictive models using artificial intelligence?
Introduction to BigProfiles as an innovative solution that enables companies to easily create powerful predictive models using artificial intelligence, even without specific skills in data science.
What are the key phases of the AI predictive model implementation process to address the churn prediction challenge?
Key phases of the AI predictive model implementation process specifically for addressing the churn prediction challenge: project definition, data collection and processing, model creation and validation.
How can the use of AI predictive models improve customer retention strategies? And what are the main challenges or ethical considerations in adopting AI-based predictive models?
Improvement of customer retention and loyalty strategies through the effective application of AI predictive models for churn prediction. Main challenges or ethical considerations to keep in mind when adopting AI-based predictive models for marketing purposes.
What are the future prospects of AI Marketing and Predictive Models in Churn Prediction?
Possible developments and future trends that could shape the role of AI and predictive models in the field of churn prediction.